My mentor, colleague, and friend Richard Bird died in April 2022 after a long battle with cancer. I wrote an obituary of him for The Guardian, his favoured newspaper; this post is a hybrid of that obituary and a eulogy I delivered at his funeral.
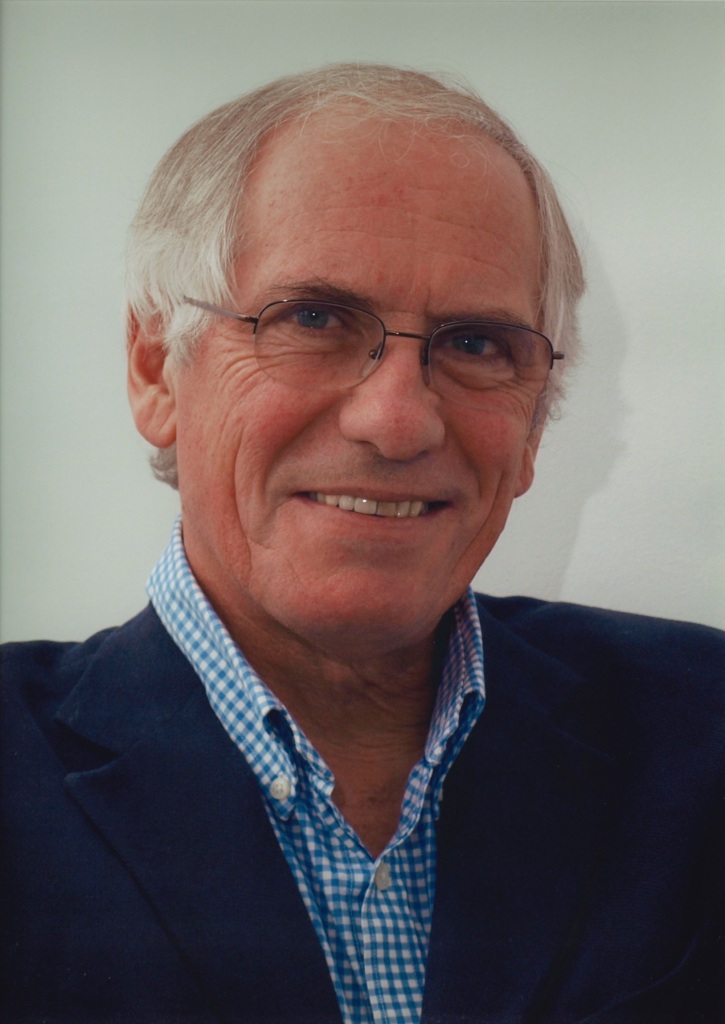
Richard was born in 1943 in London. His parents Kay and Jack were landlords of a series of pubs in South London. Richard attended St Olave’s Grammar School, then matriculated to read mathematics at Cambridge in 1960. After graduation, he had a brief spell working in sales for International Computers and Tabulators, the mainframe computer company that eventually became ICL. He was evidently effective in this role, being as personable as he was; but it involved flying around the country in small planes, which Richard did not enjoy at all. No amount of money or approval could persuade him to stay, so he left after a year to do postgraduate study at the University of London Institute of Computer Science.
Richard met Norma Lapworth at a friend’s 21st birthday party. Norma trained as an ecologist, later becoming a teacher, education advisor, and Ofsted schools inspector. Richard and Norma married in 1967.
After a year lecturing in Vancouver 1971-1972, Richard and Norma returned to the UK for Richard to take up a lectureship at the University of Reading. He was given the time finally to complete his PhD thesis, on Computational Complexity on Register Machines, in 1973. But Reading was a rather linguistically oriented department, which did not suit Richard’s mathematical temperament. So he leapt at the opportunity to join Tony Hoare’s expanding Programming Research Group at Oxford University Computing Laboratory in 1983. He was appointed as a University Lecturer in Computation, with a non-tutorial fellowship at St Cross College, assuming responsibility as Director of the MSc in Computation. He moved to a Tutorial Fellowship at Lincoln College in 1988. Richard stayed at the PRG for the remainder of his career, being promoted to Reader in Computation in 1990 and then to Professor in 1996, serving as Director of OUCL from 1998 to 2003, and finally retiring in 2008 after 25 years.
Richard’s research area was functional programming—an approach to computer programming centred around obeying the conventions of traditional mathematics. To Richard, it was self-evident that programs are mathematical entities, and that you can manipulate them in precisely the same way as you do quadratic equations. No immediate need for consideration of the capabilities of computer hardware; no entanglement in the vagaries of specific programming languages. Just algebra, pure and simple.
His PhD thesis in the early 1970s was already concerned with program transformation: the rules by which one program is shown to be equivalent to another. He wasn’t so interested in the program you actually end up with, so much as the process by which you got there, and how you know you ended up in the right place, and what alternative directions you didn’t follow en route. Specifically, he was interested in the calculus of equivalences with which you could conduct equational reasoning on programs, just as in high-school algebra.
In 1980 he was invited to participate in IFIP Working Group 2.1 on Algorithmic Languages and Calculi. This was the group that had designed the seminal programming language Algol68, and that continues to research into notations and techniques for designing and describing algorithms today. Richard had a very fruitful collaboration in WG2.1 with Lambert Meertens from the CWI at Amsterdam, developing what came to be known as the Bird-Meertens Formalism, or Squiggol to its friends. This was a concise and somewhat cryptic algebraic program notation with a powerful accompanying corpus of theorems, supporting the equational reasoning style that Richard sought.
Richard is known and respected around the world for his elegant writing. He published about 100 scientific papers in his life—not especially prolific for a scientist, but every one of those papers was very well polished. He also wrote or co-wrote seven books, starting with Programs and Machines (1976), which featured among other constructions a machine called NoRMa, the Number-Theoretic Register Machine.
I won’t go through all seven books, much as I would like to; I will just pick out a few highlights. Richard’s second book, Introduction to Functional Programming (1988, with Phil Wadler), set out his vision for teaching functional programming. Many people love this book. Graham Hutton wrote to me to say:
Richard set the standards for elegance and clarity, and the original Bird & Wadler is my all-time favourite book. It still stands up today as one of the best programming books ever written—every page is a gem.
Richard’s next book, Algebra of Programming (1996, with Oege de Moor), was much more research-oriented, extending his Squiggol agenda from functions to relations. Tim Sears tweeted:
This book blew my mind regarding what the relationship could be between math, programming and systems. I never met Richard, but his work has had a profound impact on me.
Richard’s fifth book Pearls of Functional Algorithm Design collected and repolished some of the Functional Pearls he had published over the years. These Pearls emulated Jon Bentley’s famous Programming Pearls column in CACM in the 1980s. Bentley explained:
Just as natural pearls grow from grains of sand that have irritated oysters, these programming pearls have grown from real problems that have irritated programmers. The programs are fun, and they teach important programming techniques and fundamental design principles.Richard established and was the founding editor of the Functional Pearls column, from the very first issue in 1991 of the Journal of Functional Programming until he retired in 2008. He characterized Functional Pearls as “polished, elegant, instructive, entertaining”. He had high standards for them: he advised reviewers to
stop reading when you get bored, or the material gets too complicated, or too much specialist knowledge is needed, or the writing is bad.He gave a keynote lecture about Pearls in 2006, where he observed that “most of them need more time in the oyster”.
Functional Pearls are the epitome of Richard’s writing style. Erik Meijer called him “the poet laureate of functional programming”. Greg Fitzgerald tweeted that
When life got hard, I’d read his pearls to settle my mind. His proofs read like poetry.Ed Kmett commented that Richard’s pearls book
is the only real clear exposition of how to think like a functional programmer that I’ve ever seen
I was honoured to be Richard’s co-author on his final book, Algorithm Design with Haskell, written during his illness and published in 2020. Working with him was always a joy.
Despite the global influence of his publications, Richard always saw teaching as his main duty, and research as something he did for relaxation. Andrew Ker thanked Richard for the way he had taught him as a first-year undergraduate—a way which Andrew has passed on to his own students, and which he now sees them repeating in turn. Andrew wrote:
I’ve come to see that passing on our knowledge to the next generation is the most important thing we do, even more than generating new knowledge through research. And the ideas and attitudes persist through the generations. Your influence and example extends far beyond what you know, and countless students are grateful for it.
My own favourite Richard story is a teaching one. Richard was giving a tutorial about algorithm analysis: how many steps does this program take—for example, something about processing hierarchical tree-shaped data? You’d expect a question about counting steps to have an answer in whole numbers; but in fact, the answer often involves logarithms. One student asked why this was. Richard thought for a moment, then said “logs come from trees”. The students broke out in laughter, and it took Richard a while to work out why. In fact, I think this was Richard’s own favourite Richard story too, because I know that he continued to tell it in tutorials for years afterwards.
I want to close by mentioning Richard’s openness, considerateness, and egalitarianism. The departmental secretaries always looked forward to him coming in, because he treated them with the same friendliness that he treated the most eminent of his colleagues. Several once-overawed students have written to me to express gratitude for his welcome—“no Herr Professor Doktor Doktor here, we are all colleagues”—and for helping them to find their own way.
Richard’s collaborator Lambert Meertens says:
His lectures were a joy to attend, because of their clarity and the entertaining delivery. But I’ll remember him at least as much for his kindness, his generosity, his warm interest in people’s wellbeing—expressed not only in words, but also deeds.Cezar Ionescu remembers being apprehensive about first meeting Richard at WG2.1—you should never meet your heroes—but says he
was exactly the way I had imagined him to be: very sharp, funny, wise. We talked about opera and Shakespeare, and fought (and won!) a hard battle for tap water with the hotel staff.
My own involvement with Richard started when I applied in 1987 to do a DPhil at Oxford, on a project that turned out to have finished by the time I arrived. I rattled around the Lab for a year, not even seeing my official supervisor. But Richard invited me to join his weekly Problem Solving Club. He took me under his wing, and I have never looked back. He became my DPhil supervisor, then some years later hired me into my current position, and I am privileged to have been able to call him my colleague and my friend. I basically owe him my whole career, and I have no words sufficient to convey my gratitude to him. I will miss him greatly.